Steady as she goes
If you'll forgive the nautical metaphor, forecasting longevity over the past few decades has proven to be anything but plain sailing. Those plotting a course with unshakable certainty have usually ended up storm-tossed and floating in a barrel. Since the future is unknowable, any methodology applied to forecasting must have uncertainty at its heart, which is why we advocate using stochastic projection models (and more than one at that).
Stochastic projection models are tricky beasts, however, with many methods of forecasting (ARIMA time series, drift models, penalty projections, bivariate projections, and others), each with different characteristics. One concern that arises from this sophistication is that of ensuring stability. Given any model must be sensitive to the data, how can we be sure it will provide stable and consistent results as the population changes year-on-year? This is not an academic question — when selecting internal models for Solvency II for instance, it is important to know in advance how stable such models are likely to be in the coming years. Volatility can occur for a number of model-specific reasons and where consistency is desired year-on-year it is something that must be tested for.
One obvious approach to stability testing is to back-test, and model selection will always incorporate an element of this. But what about the unknowable future? Well, it turns out that by providing an inherent statement of uncertainty, a stochastic model can provide a framework for its own future stability test. Most stochastic models can use the uncertainty measures to generate sample paths, which are simulations of future mortality, easily expressed as a grid of mortality rates by age and year. By using these mortality rates to simulate death counts which are then applied to known exposures to create future exposures, each sample path allows us to add an additional year of simulated data to the population upon which the projection was originally based.
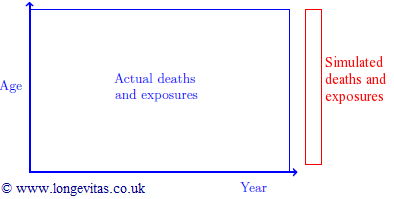
By re-running our projection against this extended dataset and examining the variability in metrics at ages of interest, we have a mechanism whereby any number of sample paths can be used to generate an equal number of stability-test projections for the coming year. You might see unwanted volatility in the metrics arising from the simulation projections, or worse, you might find simulation projections fail under certain data conditions (both compelling reasons to revisit your model). However, where these issues do not arise you extract a strong sense of comfort that your model is both robust, and, ahem, seaworthy.
Add new comment